Indigenous data sovereignty (IDS) is defined as the right of an Indigenous nation to govern the collection, ownership, and application of data generated by its members. […]
Indigenous Data Sovereignty and Blockchain
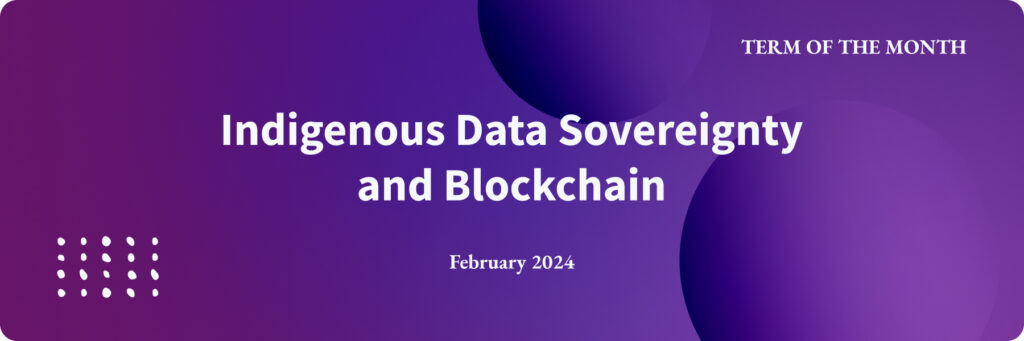
Indigenous data sovereignty (IDS) is defined as the right of an Indigenous nation to govern the collection, ownership, and application of data generated by its members. […]
Engineering Learning and Implementing Code Tests An Intern’s Perspective Nada Haboudal January 18, 2024 Nada Haboudal | January 18, 2024 Welcome to my blog post, where I showcase my work […]
Term of the Month Precision Medicine James Tabery December 8, 2023 James Tabery | December 8, 2023 Every month, ETAI will be sharing a term or concept of the month […]
Dr. Jiang explored how a groundbreaking discovery was utilized to generate 30 metadata-based features through machine learning for the automatic detection of PHI fields in structured Electronic Health Record (EHR) data. […]
Dr. Salimi offered insights for data management, machine learning, and responsible data science, emphasizing the significance of handling selection bias in algorithmic decision-making. […]
Read More… from Dr. Babak Salimi: Certifying Fair Predictive Models in the Face of Selection Bias
This term reflects the paper’s emphasis on using high-throughput machine learning models to precisely detect sensitive data, specifically Protected Health Information (PHI), in electronic health records. […]
In today’s digital age, algorithmic decision-making systems play a crucial role in fields like credit scoring and medical diagnoses. While they are often praised for being ‘objective,’ these systems can exhibit biases, mostly stemming from the data they rely on. […]
Dr. Malin drew upon examples from large-scale data-driven projects like the EMR and bio-repository at Vanderbilt University Medical Center, the eMERGE consortium of the NIH, and the All of Us Research Program, aiming to create a comprehensive database of EMRs, genome sequences, and mHealth records from one million Americans. […]
“Dignitary privacy is based on a belief that privacy is intrinsically valuable, whereas resource privacy is based on a belief that privacy is simply a tool that has instrumental value.”
Hughes, RL David. […]
Dr Mathews provided an overview of the recent NASEM consensus study report that focused on the use of race and ethnicity and other population descriptors in genomics research, including the recommendations made by the committee. […]