Dr. Tim Mackey: Leveraging Blockchain Technology to Enable Indigenous Data Sovereignty of Genomic Data
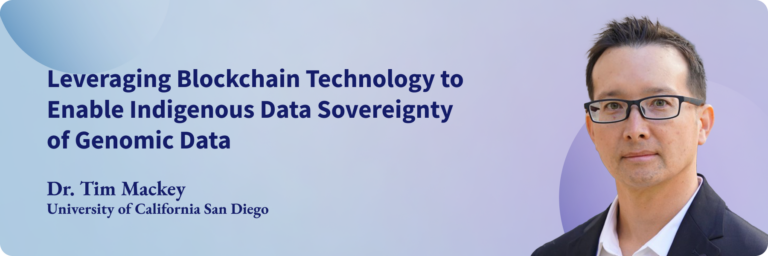
Dr. Mackey discussed a project funded by the Robert Wood Johnson Foundation in partnership with The Native Biodata Consortium to develop a blockchain-based governance system for managing Indigenous genomic data.